AGN-host decomposition and the quest for dual AGNs with Euclid first light (in prep.)
Analysis of the AGN host-galaxies in the Euclid Early Release
Observations, with particular focus on the Perseus Cluster.
Development of a new software, GingaFit (https://github.com/
AstroFederica/GingaFit/tree/main), devoted to AGN-host
decomposition and the detection of dual and binary
supermassive back holes. Preliminary results were presented
in the publication by Cuillandre et al., 2024, Euclid: Early
Release Observations – Overview of the Perseus cluster and
analysis of its luminosity and stellar mass functions, showcased
in the ESA Euclid Press release on May 2024.
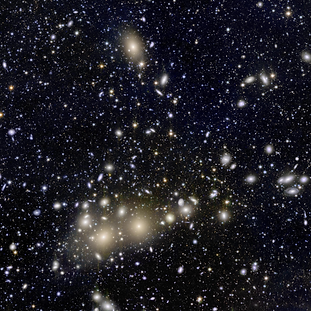
Courtesy of Jean-Charles Cuillandre
Preliminary

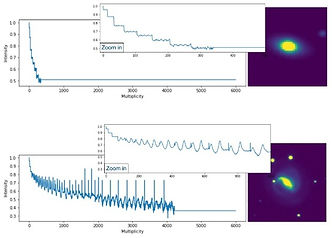_edited_edited_ed.jpg)
Image feature extraction and galaxy classification: a novel and efficient approach with automated machine learning.
In this work we explore the possibility of applying machine learning methods designed for one-dimensional problems to the task of galaxy image classification. The algorithms used for image classification typically rely on multiple costly steps, such as the Point Spread Function (PSF) deconvolution and the training and application of complex Convolutional Neural Networks (CNN) of thousands or even millions of parameters. In our approach, we extract features from the galaxy images by analysing the elliptical isophotes in their light distribution and collect the information in a sequence. The sequences obtained with this method present definite features allowing a direct distinction between galaxy types, as opposed to smooth Sérsic profiles. Then, we train and classify the sequences with machine learning algorithms, designed through the platform Modulos AutoML, and study how they optimize the classification task. As a demonstration of this method, we use the second public release of the Dark Energy Survey (DES DR2). We show that by applying it to this sample we are able to successfully distinguish between early-type and late-type galaxies, for images with signal-to-noise ratio greater then 300. This yields an accuracy of 86% for the early-type galaxies and 93% for the late-type galaxies, which is on par with most contemporary automated image classification approaches. Our novel method allows for galaxy images to be accurately classified and is faster than other approaches. Data dimensionality reduction also implies a significant lowering in computational cost. In the perspective of future data sets obtained with e.g. Euclid and the Vera Rubin Observatory (VRO), this work represents a path towards using a well-tested and widely used platform from industry in efficiently tackling galaxy classification problems at the peta-byte scale.
Predicting Cosmological Observables with PyCosmo
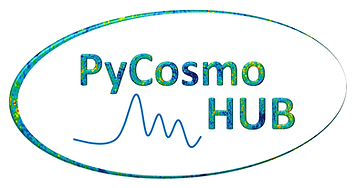
Current and upcoming cosmological experiments open a new era of precision cosmology, thus demanding accurate theoretical predictions for cosmological observables. Because of the complexity of the codes delivering such predictions, reaching a high level of numerical accuracy is challenging. Among the codes already fulfilling this task, 𝖯𝗒𝖢𝗈𝗌𝗆𝗈 is a Python based framework providing solutions to the Einstein-Boltzmann equations and accurate predictions for cosmological observables. In this work, we first describe how the observables are implemented. Then, we check the accuracy of the theoretical predictions for background quantities, power spectra and Limber and beyond-Limber angular power spectra by comparison with other codes: the Core Cosmology Library (𝙲𝙲𝙻), 𝙲𝙻𝙰𝚂𝚂, 𝙷𝙼𝙲𝚘𝚍𝚎 and 𝚒𝙲𝚘𝚜𝚖𝚘. In our analysis we quantify the agreement of 𝖯𝗒𝖢𝗈𝗌𝗆𝗈 with the other codes, for a range of cosmological models, monitored through a series of unit tests. 𝖯𝗒𝖢𝗈𝗌𝗆𝗈, conceived as a multi purpose cosmology calculation tool in 𝙿𝚢𝚝𝚑𝚘𝚗, is designed to be interactive and user friendly. A current version of the code (without the Boltzmann Solver) is publicly available and can be used interactively on the platform 𝖯𝗒𝖢𝗈𝗌𝗆𝗈 𝖧𝗎𝖻, all accessible from this link: this https URL . On the hub the users can perform their own computations using 𝙹𝚞𝚙𝚢𝚝𝚎𝚛 𝙽𝚘𝚝𝚎𝚋𝚘𝚘𝚔𝚜 without the need of installing any software, access to the results presented in this work and benefit from tutorial notebooks illustrating the usage of the code. The link above also redirects to the code release and documentation.
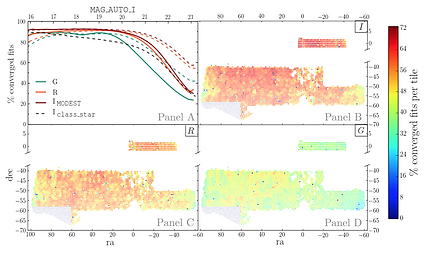
A catalogue of structural and morphological measurements for DES Y1
We present a structural and morphological catalogue for 45 million objects selected from the first year of data from the Dark Energy Survey (DES). Single Sersic fits and non-parametric measurements are produced for g, r and i filters. The parameters from the best-fitting Sersic model (total magnitude, half-light radius, Sersic index, axis ratio and position angle) are measured with Galfit; the non-parametric coefficients (concentration, asymmetry, clumpiness, Gini, M20) are provided using the Zurich Estimator of Structural Types (ZEST+). To study the statistical uncertainties, we consider a sample of state-of-the-art image simulations with a realistic distribution in the input parameter space and then process and analyse them as we do with real data: this enables us to quantify the observational biases due to PSF blurring and magnitude effects and correct the measurements as a function of magnitude, galaxy size, Sersic index (concentration for the analysis of the non-parametric measurements) and ellipticity. We present the largest structural catalogue to date: we find that accurate and complete measurements for all the structural parameters are typically obtained for galaxies with SExtractor MAG AUTO I < 21. Indeed, the parameters in the filters i and r can be overall well recovered up to MAG AUTO < 21.5, corresponding to a fitting completeness of ~90% below this threshold, for a total of 25 million galaxies. The combination of parametric and non-parametric structural measurements makes this catalogue an important instrument to explore and understand how galaxies form and evolve. The catalogue described in this paper was publicly released alongside the Dark Energy Survey collaboration Y1 cosmology data products.
Collaborative work: Evidence for Dynamically Driven Formation of the GW170817 Neutron Star Binary in NGC 4993
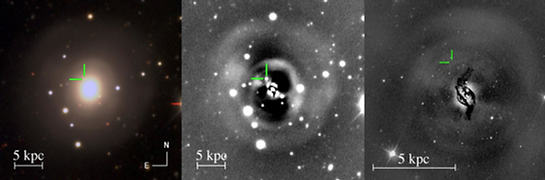
The tools developed for the production of the galaxy morphology catalogue for DES Y1, which is one of the main subjects of this thesis, and the consequent knowledge acquired about DES photometric data, guided the structural analysis of NGC 4993, known also as the host galaxy of the GW170817 gravitational wave event, generated by a neutron star binary system. A complete study of the galaxy star-formation history and morphology is reported in \cite{Palmese}. The morphological analysis was made in terms of multi-wavelength parametric fits and non-parametric analyses, whose combined information was able to give important insights on the properties and evolutionary history of this shell-like galaxy. The values found for Concentration, Clumpiness and Asymmetry are in line with an ETG. The results from the parametric fitting reveal aspects of the galaxy sub-structure. More precisely, it reported increasing values for the Sérsic index towards redder bands and different values in the position angle, suggesting rotation of bluer versus redder wavelengths. This might indicate the presence of two superimposed stellar populations with different orientations. The overposition may have arisen from a galaxy merger, as also suggested by the presence of galaxy shells. The shell structure is clearly visible in the residuals of the parametric fits, as shown in figure above (and reported in Figure 1 of the original paper). The authors used the evidences collected from the structural analysis and spectroscopic fitting to sustain the scenario that there is a relation between the dynamical interactions occurred during a galaxy merger and the formation of the neutron star binary that produced the gravitational wave signal.